Phase Imaging with Computational Specificity
— Digital staining for correlative imaging without fluorescence
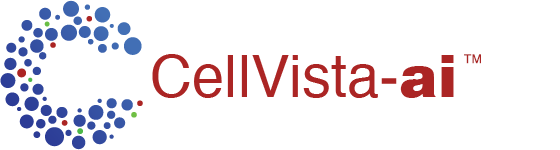
In the absence of labels, QPI (Quantitative Phase Imaging) cannot easily identify particular structures in the cell, i.e. no specificity.
Artificial intelligence (AI) can map one form of contrast into another, a concept coined as image-to-image translation: a deep neural network (DNN) can predict the mapping of a stain or fluorescence marker from label-free QPI images as input. Using this concept, the Popescu group at UIUC Beckman Institute developed Phase Imaging with Computational Specificity (PICS): a neural network learns from label-free (CellVista SLIM or CellVista GLIM) and ground-truth fluorescence images to predict where specific fluorophores would bind in an unlabelled specimen – thus providing digital staining (label-free) of the QPI quantitative image, i.e. PICS.
The digital staining masks are used to segment QPI (SLIM or GLIM) images to extract dry mass, morphology and refractive index values for 2D and 3D quantitative assays. Even more, the neural network can extract cell cycle-, cell viability-dependent (and more) features from the nanoscale structure measured in QPI images. 2D and 3D assays can be performed with the accuracy and specificity of regular fluorescence, but without the inconveniences associated with phototoxicity and photobleaching.
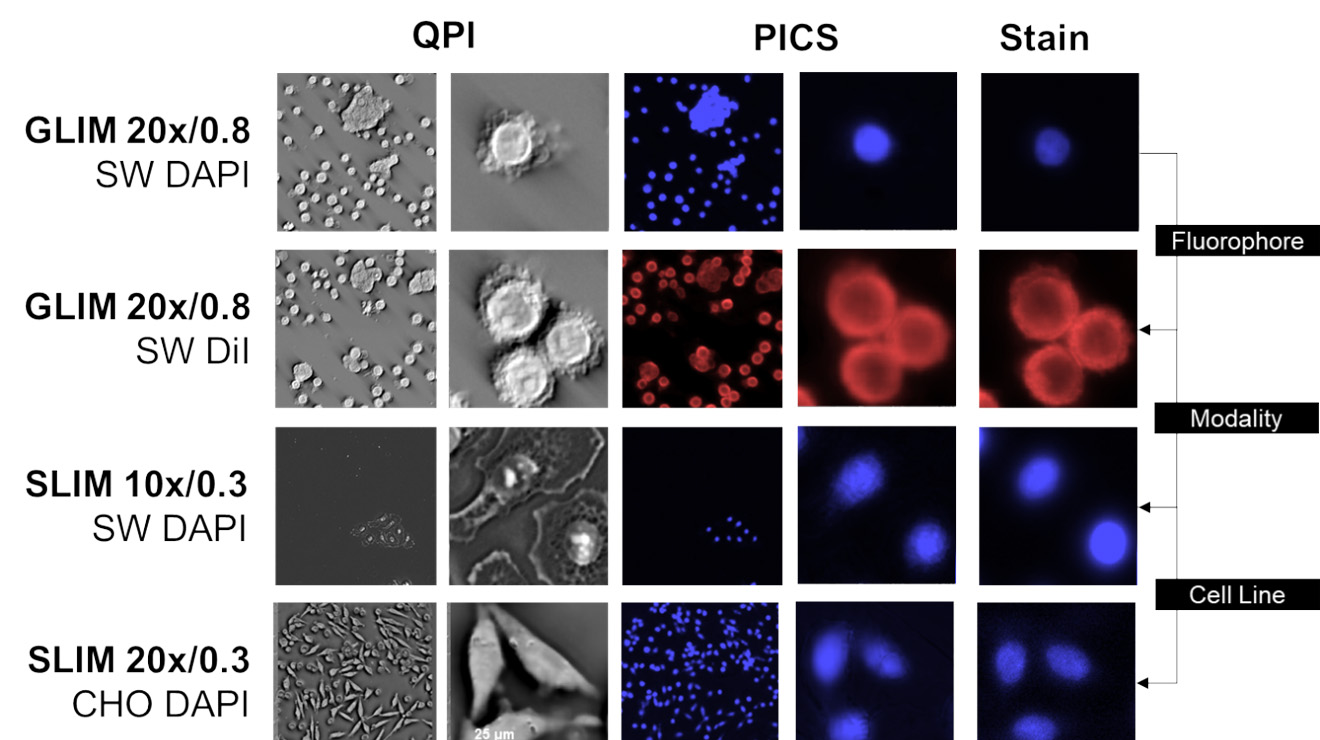
QPI PICS prediction: blue – nucleus, pink - cytoplasm (with permission from Kandel ME et al, Nat Commun. 2020)